In today’s digital landscape, the pace of advancement in artificial intelligence (AI) is unprecedented. As organizations adopt AI technologies to streamline operations and enhance customer experiences, the function of Quality Assurance (QA) is evolving significantly. While AI offers remarkable opportunities, it also poses challenges that demand a refined approach to QA. Let’s explore how this shift is impacting QA professionals and the actions they can take to thrive in this new environment.
The Shifting Landscape of Quality Assurance
Historically, QA's role was to identify bugs and issues late in the development process. This approach often led to last-minute fixes and, ultimately, dissatisfaction from users. Now, QA is more proactive, becoming part of the project from the very beginning. A staggering 70% of issues can be identified and resolved during the design phase, significantly reducing time and costs in later stages.
QA professionals must now work alongside developers, embedding quality into every step of a project. This proactive approach encourages teams to create better products. For example, when using feedback from QA early in the development process, companies can reduce post-release bug reports by over 50%.
The Integration of AI in Testing
AI is redefining how QA teams operate. Automation has emerged as a cornerstone of modern QA practices, and integrating AI helps enhance automation's effectiveness. AI tools can analyze large datasets quickly, apply predictive analytics, and pinpoint potential defects faster than traditional methods.
Instead of routine tasks, QA teams can focus on complex testing scenarios that require human input. For example, AI can generate tailored test cases based on actual user behavior, improving the relevance of quality checks. According to industry studies, this approach can increase testing efficiency by 40%, allowing teams to deploy updates more frequently and reliably.
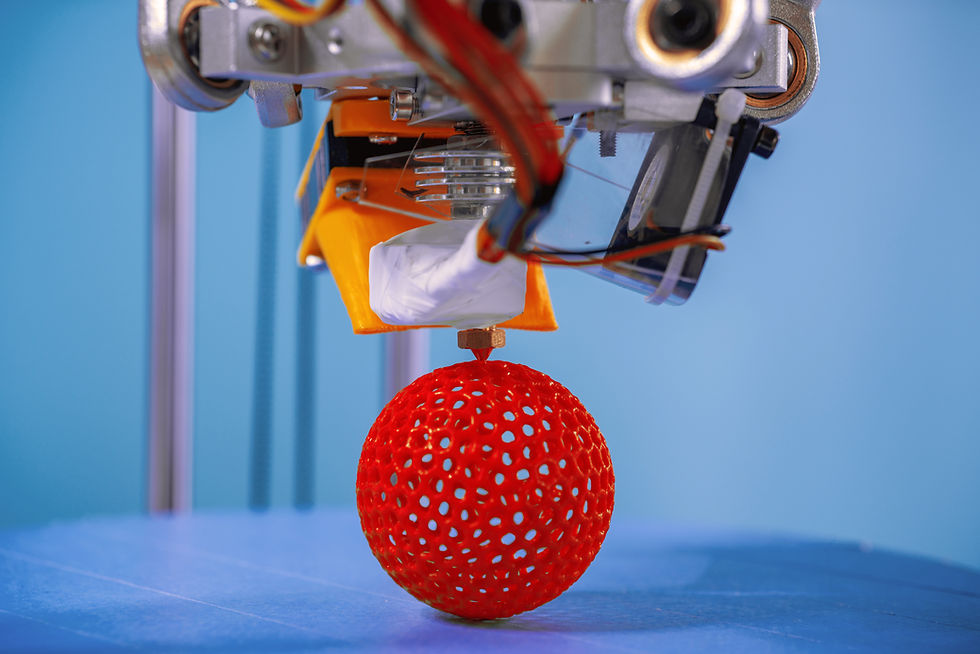
Challenging Preconceived Notions
Though AI tools promise to streamline the QA process, they also introduce new challenges. Quality can be compromised if AI systems are trained on biased datasets. If, for example, an AI tool was trained on data lacking diversity, it might fail to recognize issues affecting certain user groups. Thus, QA professionals must be vigilant about ensuring the integrity of both the data and the algorithms used.
The human element remains critical in QA. While AI handles repetitive tasks efficiently, human insight is necessary for understanding user needs and ethical considerations. For instance, during the testing of a virtual assistant, human testers can assess user satisfaction and empathy in ways that machines cannot. Balancing AI tools with human insights ensures better user experiences.
New Skill Sets for QA Professionals
As the QA landscape evolves, professionals need to develop new skills. Familiarity with AI and machine learning is no longer optional—it is essential. According to a recent survey, 67% of QA professionals indicate they are currently learning programming languages like Python or frameworks such as TensorFlow to keep up with industry needs.
Data analysis skills are also critical. QA professionals should be able to interpret data and extract actionable insights. Being adept in analytics allows teams to refine their testing strategies and achieve consistently high-quality results. Those willing to adapt will find exciting opportunities in a rapidly changing environment.
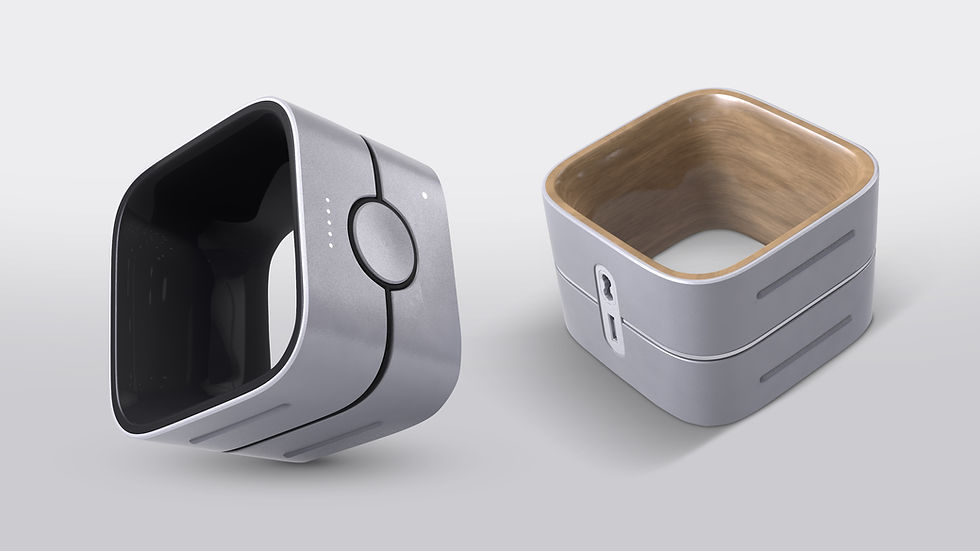
Collaborative Dynamics with Development Teams
For QA teams to maximize AI's potential, close collaboration with development teams is essential. By breaking down barriers and fostering a culture of shared accountability, both teams can enhance quality outcomes. Communicating openly facilitates quicker iterations and more effective solutions, yielding better products.
Using agile methodologies alongside AI testing tools not only accelerates release cycles but preserves high quality. Companies employing this collaborative approach report up to a 30% reduction in time-to-market for software releases.
Future Trends in AI and QA
Several trends will shape the future of QA as AI becomes more integrated into testing. One significant trend is ‘shift-left’ testing, which promotes QA activities at the outset of development. Doing this helps organizations catch issues early, reducing development costs by up to 30% and facilitating quicker deployments.
Additionally, autonomous testing tools that adapt and learn from past tests are becoming more prevalent. Over time, these tools will enhance their efficiency and accuracy, making them invaluable for QA teams. As technology continues to evolve, ongoing education and adaptation will be essential for success.
Embracing Change and Looking Ahead
Navigating the complexities of an AI-driven world necessitates a proactive approach. QA professionals must embrace change, continually seeking to learn new skills and adapt to innovative tools. As companies increasingly prioritize quality and user satisfaction, the role of QA will become ever more critical.
The future of QA in an AI-centric landscape is one filled with opportunity. By remaining flexible and focused on learning, QA professionals can lead their teams through the challenges and embrace the opportunities presented by AI. This evolution not only ensures higher software quality but also guarantees better user experiences in an increasingly digital world.
Stay Updated and Keep Learning
To stay ahead in the ever-changing QA landscape, consider:
Enrolling in AI-focused QA courses (Explore Courses)
Joining a QA community
Keeping up with QA trends and best practices (Read More)
What AI-powered testing tools has your team implemented?
Opmerkingen